File size: 29,485 Bytes
20dfaad |
1 2 3 4 5 6 7 8 9 10 11 12 13 14 15 16 17 18 19 20 21 22 23 24 25 26 27 28 29 30 31 32 33 34 35 36 37 38 39 40 41 42 43 44 45 46 47 48 49 50 51 52 53 54 55 56 57 58 59 60 61 62 63 64 65 66 67 68 69 70 71 72 73 74 75 76 77 78 79 80 81 82 83 84 85 86 87 88 89 90 91 92 93 94 95 96 97 98 99 100 101 102 103 104 105 106 107 108 109 110 111 112 113 114 115 116 117 118 119 120 121 122 123 124 125 126 127 128 129 130 131 132 133 134 135 136 137 138 139 140 141 142 143 144 145 146 147 148 149 150 151 152 153 154 155 156 157 158 159 160 161 162 163 164 165 166 167 168 169 170 171 172 173 174 175 176 177 178 179 180 181 182 183 184 185 186 187 188 189 190 191 192 193 194 195 196 197 198 199 200 201 202 203 204 205 206 207 208 209 210 211 212 213 214 215 216 217 218 219 220 221 222 223 224 225 226 227 228 229 230 231 232 233 234 235 236 237 238 239 240 241 242 243 244 245 246 247 248 249 250 251 252 253 254 255 256 257 258 259 260 261 262 263 264 265 266 267 268 269 270 271 272 273 274 275 276 277 278 279 280 281 282 283 284 285 286 287 288 289 290 291 292 293 294 295 296 297 298 299 300 301 302 303 304 305 306 307 308 309 310 311 312 313 314 315 316 317 318 319 320 321 322 323 324 325 326 327 328 329 330 331 332 333 334 335 336 337 338 339 340 341 342 343 344 345 346 347 348 349 350 351 352 353 354 355 356 357 358 359 360 361 362 363 364 365 366 367 368 369 370 371 372 373 374 375 376 377 378 379 380 381 382 383 384 385 386 387 388 389 390 391 392 393 394 395 396 397 398 399 400 401 402 403 404 405 406 407 408 409 410 411 412 413 414 415 416 417 418 419 420 421 422 423 424 425 426 427 428 429 430 431 432 433 434 435 436 437 438 439 440 441 442 443 444 445 446 447 448 449 450 451 452 453 454 455 456 457 458 459 460 461 462 463 464 465 466 467 468 469 470 471 472 473 474 475 476 477 478 479 480 481 482 483 484 485 486 487 488 489 490 491 492 493 494 495 496 497 498 499 500 501 502 503 504 505 506 507 508 509 510 511 512 513 514 515 516 517 518 519 520 521 522 523 524 525 526 527 528 529 530 531 532 533 534 535 536 537 538 539 540 541 542 543 544 545 546 547 548 549 550 551 552 553 554 555 556 557 558 559 560 561 562 563 564 565 566 567 568 569 570 571 572 573 574 575 576 577 578 579 580 581 582 583 |
---
base_model: Herman555/dolphin-2.2.1-AshhLimaRP-Mistral-7B-GGUF
inference: false
license: apache-2.0
model_creator: Yamam
model_name: Dolphin 2.2.1 AshhLimaRP Mistral 7B
model_type: mistral
prompt_template: '<|im_start|>system
{system_message}<|im_end|>
<|im_start|>user
{prompt}<|im_end|>
<|im_start|>assistant
'
quantized_by: TheBloke
tags:
- not-for-all-audiences
---
<!-- markdownlint-disable MD041 -->
<!-- header start -->
<!-- 200823 -->
<div style="width: auto; margin-left: auto; margin-right: auto">
<img src="https://i.imgur.com/EBdldam.jpg" alt="TheBlokeAI" style="width: 100%; min-width: 400px; display: block; margin: auto;">
</div>
<div style="display: flex; justify-content: space-between; width: 100%;">
<div style="display: flex; flex-direction: column; align-items: flex-start;">
<p style="margin-top: 0.5em; margin-bottom: 0em;"><a href="https://discord.gg/theblokeai">Chat & support: TheBloke's Discord server</a></p>
</div>
<div style="display: flex; flex-direction: column; align-items: flex-end;">
<p style="margin-top: 0.5em; margin-bottom: 0em;"><a href="https://www.patreon.com/TheBlokeAI">Want to contribute? TheBloke's Patreon page</a></p>
</div>
</div>
<div style="text-align:center; margin-top: 0em; margin-bottom: 0em"><p style="margin-top: 0.25em; margin-bottom: 0em;">TheBloke's LLM work is generously supported by a grant from <a href="https://a16z.com">andreessen horowitz (a16z)</a></p></div>
<hr style="margin-top: 1.0em; margin-bottom: 1.0em;">
<!-- header end -->
# Dolphin 2.2.1 AshhLimaRP Mistral 7B - GGUF
- Model creator: [Yamam](https://huggingface.co/Herman555)
- Original model: [Dolphin 2.2.1 AshhLimaRP Mistral 7B](https://huggingface.co/Herman555/dolphin-2.2.1-AshhLimaRP-Mistral-7B-GGUF)
<!-- description start -->
## Description
This repo contains GGUF format model files for [Yamam's Dolphin 2.2.1 AshhLimaRP Mistral 7B](https://huggingface.co/Herman555/dolphin-2.2.1-AshhLimaRP-Mistral-7B-GGUF).
These files were quantised using hardware kindly provided by [Massed Compute](https://massedcompute.com/).
<!-- description end -->
<!-- README_GGUF.md-about-gguf start -->
### About GGUF
GGUF is a new format introduced by the llama.cpp team on August 21st 2023. It is a replacement for GGML, which is no longer supported by llama.cpp.
Here is an incomplete list of clients and libraries that are known to support GGUF:
* [llama.cpp](https://github.com/ggerganov/llama.cpp). The source project for GGUF. Offers a CLI and a server option.
* [text-generation-webui](https://github.com/oobabooga/text-generation-webui), the most widely used web UI, with many features and powerful extensions. Supports GPU acceleration.
* [KoboldCpp](https://github.com/LostRuins/koboldcpp), a fully featured web UI, with GPU accel across all platforms and GPU architectures. Especially good for story telling.
* [LM Studio](https://lmstudio.ai/), an easy-to-use and powerful local GUI for Windows and macOS (Silicon), with GPU acceleration.
* [LoLLMS Web UI](https://github.com/ParisNeo/lollms-webui), a great web UI with many interesting and unique features, including a full model library for easy model selection.
* [Faraday.dev](https://faraday.dev/), an attractive and easy to use character-based chat GUI for Windows and macOS (both Silicon and Intel), with GPU acceleration.
* [ctransformers](https://github.com/marella/ctransformers), a Python library with GPU accel, LangChain support, and OpenAI-compatible AI server.
* [llama-cpp-python](https://github.com/abetlen/llama-cpp-python), a Python library with GPU accel, LangChain support, and OpenAI-compatible API server.
* [candle](https://github.com/huggingface/candle), a Rust ML framework with a focus on performance, including GPU support, and ease of use.
<!-- README_GGUF.md-about-gguf end -->
<!-- repositories-available start -->
## Repositories available
* [AWQ model(s) for GPU inference.](https://huggingface.co/TheBloke/dolphin-2.2.1-AshhLimaRP-Mistral-7B-AWQ)
* [GPTQ models for GPU inference, with multiple quantisation parameter options.](https://huggingface.co/TheBloke/dolphin-2.2.1-AshhLimaRP-Mistral-7B-GPTQ)
* [2, 3, 4, 5, 6 and 8-bit GGUF models for CPU+GPU inference](https://huggingface.co/TheBloke/dolphin-2.2.1-AshhLimaRP-Mistral-7B-GGUF)
* [Yamam's original unquantised fp16 model in pytorch format, for GPU inference and for further conversions](https://huggingface.co/Herman555/dolphin-2.2.1-AshhLimaRP-Mistral-7B-GGUF)
<!-- repositories-available end -->
<!-- prompt-template start -->
## Prompt template: ChatML
```
<|im_start|>system
{system_message}<|im_end|>
<|im_start|>user
{prompt}<|im_end|>
<|im_start|>assistant
```
<!-- prompt-template end -->
<!-- compatibility_gguf start -->
## Compatibility
These quantised GGUFv2 files are compatible with llama.cpp from August 27th onwards, as of commit [d0cee0d](https://github.com/ggerganov/llama.cpp/commit/d0cee0d36d5be95a0d9088b674dbb27354107221)
They are also compatible with many third party UIs and libraries - please see the list at the top of this README.
## Explanation of quantisation methods
<details>
<summary>Click to see details</summary>
The new methods available are:
* GGML_TYPE_Q2_K - "type-1" 2-bit quantization in super-blocks containing 16 blocks, each block having 16 weight. Block scales and mins are quantized with 4 bits. This ends up effectively using 2.5625 bits per weight (bpw)
* GGML_TYPE_Q3_K - "type-0" 3-bit quantization in super-blocks containing 16 blocks, each block having 16 weights. Scales are quantized with 6 bits. This end up using 3.4375 bpw.
* GGML_TYPE_Q4_K - "type-1" 4-bit quantization in super-blocks containing 8 blocks, each block having 32 weights. Scales and mins are quantized with 6 bits. This ends up using 4.5 bpw.
* GGML_TYPE_Q5_K - "type-1" 5-bit quantization. Same super-block structure as GGML_TYPE_Q4_K resulting in 5.5 bpw
* GGML_TYPE_Q6_K - "type-0" 6-bit quantization. Super-blocks with 16 blocks, each block having 16 weights. Scales are quantized with 8 bits. This ends up using 6.5625 bpw
Refer to the Provided Files table below to see what files use which methods, and how.
</details>
<!-- compatibility_gguf end -->
<!-- README_GGUF.md-provided-files start -->
## Provided files
| Name | Quant method | Bits | Size | Max RAM required | Use case |
| ---- | ---- | ---- | ---- | ---- | ----- |
| [dolphin-2.2.1-ashhlimarp-mistral-7b.Q2_K.gguf](https://huggingface.co/TheBloke/dolphin-2.2.1-AshhLimaRP-Mistral-7B-GGUF/blob/main/dolphin-2.2.1-ashhlimarp-mistral-7b.Q2_K.gguf) | Q2_K | 2 | 3.08 GB| 5.58 GB | smallest, significant quality loss - not recommended for most purposes |
| [dolphin-2.2.1-ashhlimarp-mistral-7b.Q3_K_S.gguf](https://huggingface.co/TheBloke/dolphin-2.2.1-AshhLimaRP-Mistral-7B-GGUF/blob/main/dolphin-2.2.1-ashhlimarp-mistral-7b.Q3_K_S.gguf) | Q3_K_S | 3 | 3.16 GB| 5.66 GB | very small, high quality loss |
| [dolphin-2.2.1-ashhlimarp-mistral-7b.Q3_K_M.gguf](https://huggingface.co/TheBloke/dolphin-2.2.1-AshhLimaRP-Mistral-7B-GGUF/blob/main/dolphin-2.2.1-ashhlimarp-mistral-7b.Q3_K_M.gguf) | Q3_K_M | 3 | 3.52 GB| 6.02 GB | very small, high quality loss |
| [dolphin-2.2.1-ashhlimarp-mistral-7b.Q3_K_L.gguf](https://huggingface.co/TheBloke/dolphin-2.2.1-AshhLimaRP-Mistral-7B-GGUF/blob/main/dolphin-2.2.1-ashhlimarp-mistral-7b.Q3_K_L.gguf) | Q3_K_L | 3 | 3.82 GB| 6.32 GB | small, substantial quality loss |
| [dolphin-2.2.1-ashhlimarp-mistral-7b.Q4_0.gguf](https://huggingface.co/TheBloke/dolphin-2.2.1-AshhLimaRP-Mistral-7B-GGUF/blob/main/dolphin-2.2.1-ashhlimarp-mistral-7b.Q4_0.gguf) | Q4_0 | 4 | 4.11 GB| 6.61 GB | legacy; small, very high quality loss - prefer using Q3_K_M |
| [dolphin-2.2.1-ashhlimarp-mistral-7b.Q4_K_S.gguf](https://huggingface.co/TheBloke/dolphin-2.2.1-AshhLimaRP-Mistral-7B-GGUF/blob/main/dolphin-2.2.1-ashhlimarp-mistral-7b.Q4_K_S.gguf) | Q4_K_S | 4 | 4.14 GB| 6.64 GB | small, greater quality loss |
| [dolphin-2.2.1-ashhlimarp-mistral-7b.Q4_K_M.gguf](https://huggingface.co/TheBloke/dolphin-2.2.1-AshhLimaRP-Mistral-7B-GGUF/blob/main/dolphin-2.2.1-ashhlimarp-mistral-7b.Q4_K_M.gguf) | Q4_K_M | 4 | 4.37 GB| 6.87 GB | medium, balanced quality - recommended |
| [dolphin-2.2.1-ashhlimarp-mistral-7b.Q5_0.gguf](https://huggingface.co/TheBloke/dolphin-2.2.1-AshhLimaRP-Mistral-7B-GGUF/blob/main/dolphin-2.2.1-ashhlimarp-mistral-7b.Q5_0.gguf) | Q5_0 | 5 | 5.00 GB| 7.50 GB | legacy; medium, balanced quality - prefer using Q4_K_M |
| [dolphin-2.2.1-ashhlimarp-mistral-7b.Q5_K_S.gguf](https://huggingface.co/TheBloke/dolphin-2.2.1-AshhLimaRP-Mistral-7B-GGUF/blob/main/dolphin-2.2.1-ashhlimarp-mistral-7b.Q5_K_S.gguf) | Q5_K_S | 5 | 5.00 GB| 7.50 GB | large, low quality loss - recommended |
| [dolphin-2.2.1-ashhlimarp-mistral-7b.Q5_K_M.gguf](https://huggingface.co/TheBloke/dolphin-2.2.1-AshhLimaRP-Mistral-7B-GGUF/blob/main/dolphin-2.2.1-ashhlimarp-mistral-7b.Q5_K_M.gguf) | Q5_K_M | 5 | 5.13 GB| 7.63 GB | large, very low quality loss - recommended |
| [dolphin-2.2.1-ashhlimarp-mistral-7b.Q6_K.gguf](https://huggingface.co/TheBloke/dolphin-2.2.1-AshhLimaRP-Mistral-7B-GGUF/blob/main/dolphin-2.2.1-ashhlimarp-mistral-7b.Q6_K.gguf) | Q6_K | 6 | 5.94 GB| 8.44 GB | very large, extremely low quality loss |
| [dolphin-2.2.1-ashhlimarp-mistral-7b.Q8_0.gguf](https://huggingface.co/TheBloke/dolphin-2.2.1-AshhLimaRP-Mistral-7B-GGUF/blob/main/dolphin-2.2.1-ashhlimarp-mistral-7b.Q8_0.gguf) | Q8_0 | 8 | 7.70 GB| 10.20 GB | very large, extremely low quality loss - not recommended |
**Note**: the above RAM figures assume no GPU offloading. If layers are offloaded to the GPU, this will reduce RAM usage and use VRAM instead.
<!-- README_GGUF.md-provided-files end -->
<!-- README_GGUF.md-how-to-download start -->
## How to download GGUF files
**Note for manual downloaders:** You almost never want to clone the entire repo! Multiple different quantisation formats are provided, and most users only want to pick and download a single file.
The following clients/libraries will automatically download models for you, providing a list of available models to choose from:
* LM Studio
* LoLLMS Web UI
* Faraday.dev
### In `text-generation-webui`
Under Download Model, you can enter the model repo: TheBloke/dolphin-2.2.1-AshhLimaRP-Mistral-7B-GGUF and below it, a specific filename to download, such as: dolphin-2.2.1-ashhlimarp-mistral-7b.Q4_K_M.gguf.
Then click Download.
### On the command line, including multiple files at once
I recommend using the `huggingface-hub` Python library:
```shell
pip3 install huggingface-hub
```
Then you can download any individual model file to the current directory, at high speed, with a command like this:
```shell
huggingface-cli download TheBloke/dolphin-2.2.1-AshhLimaRP-Mistral-7B-GGUF dolphin-2.2.1-ashhlimarp-mistral-7b.Q4_K_M.gguf --local-dir . --local-dir-use-symlinks False
```
<details>
<summary>More advanced huggingface-cli download usage</summary>
You can also download multiple files at once with a pattern:
```shell
huggingface-cli download TheBloke/dolphin-2.2.1-AshhLimaRP-Mistral-7B-GGUF --local-dir . --local-dir-use-symlinks False --include='*Q4_K*gguf'
```
For more documentation on downloading with `huggingface-cli`, please see: [HF -> Hub Python Library -> Download files -> Download from the CLI](https://huggingface.co/docs/huggingface_hub/guides/download#download-from-the-cli).
To accelerate downloads on fast connections (1Gbit/s or higher), install `hf_transfer`:
```shell
pip3 install hf_transfer
```
And set environment variable `HF_HUB_ENABLE_HF_TRANSFER` to `1`:
```shell
HF_HUB_ENABLE_HF_TRANSFER=1 huggingface-cli download TheBloke/dolphin-2.2.1-AshhLimaRP-Mistral-7B-GGUF dolphin-2.2.1-ashhlimarp-mistral-7b.Q4_K_M.gguf --local-dir . --local-dir-use-symlinks False
```
Windows Command Line users: You can set the environment variable by running `set HF_HUB_ENABLE_HF_TRANSFER=1` before the download command.
</details>
<!-- README_GGUF.md-how-to-download end -->
<!-- README_GGUF.md-how-to-run start -->
## Example `llama.cpp` command
Make sure you are using `llama.cpp` from commit [d0cee0d](https://github.com/ggerganov/llama.cpp/commit/d0cee0d36d5be95a0d9088b674dbb27354107221) or later.
```shell
./main -ngl 32 -m dolphin-2.2.1-ashhlimarp-mistral-7b.Q4_K_M.gguf --color -c 2048 --temp 0.7 --repeat_penalty 1.1 -n -1 -p "<|im_start|>system\n{system_message}<|im_end|>\n<|im_start|>user\n{prompt}<|im_end|>\n<|im_start|>assistant"
```
Change `-ngl 32` to the number of layers to offload to GPU. Remove it if you don't have GPU acceleration.
Change `-c 2048` to the desired sequence length. For extended sequence models - eg 8K, 16K, 32K - the necessary RoPE scaling parameters are read from the GGUF file and set by llama.cpp automatically.
If you want to have a chat-style conversation, replace the `-p <PROMPT>` argument with `-i -ins`
For other parameters and how to use them, please refer to [the llama.cpp documentation](https://github.com/ggerganov/llama.cpp/blob/master/examples/main/README.md)
## How to run in `text-generation-webui`
Further instructions here: [text-generation-webui/docs/llama.cpp.md](https://github.com/oobabooga/text-generation-webui/blob/main/docs/llama.cpp.md).
## How to run from Python code
You can use GGUF models from Python using the [llama-cpp-python](https://github.com/abetlen/llama-cpp-python) or [ctransformers](https://github.com/marella/ctransformers) libraries.
### How to load this model in Python code, using ctransformers
#### First install the package
Run one of the following commands, according to your system:
```shell
# Base ctransformers with no GPU acceleration
pip install ctransformers
# Or with CUDA GPU acceleration
pip install ctransformers[cuda]
# Or with AMD ROCm GPU acceleration (Linux only)
CT_HIPBLAS=1 pip install ctransformers --no-binary ctransformers
# Or with Metal GPU acceleration for macOS systems only
CT_METAL=1 pip install ctransformers --no-binary ctransformers
```
#### Simple ctransformers example code
```python
from ctransformers import AutoModelForCausalLM
# Set gpu_layers to the number of layers to offload to GPU. Set to 0 if no GPU acceleration is available on your system.
llm = AutoModelForCausalLM.from_pretrained("TheBloke/dolphin-2.2.1-AshhLimaRP-Mistral-7B-GGUF", model_file="dolphin-2.2.1-ashhlimarp-mistral-7b.Q4_K_M.gguf", model_type="mistral", gpu_layers=50)
print(llm("AI is going to"))
```
## How to use with LangChain
Here are guides on using llama-cpp-python and ctransformers with LangChain:
* [LangChain + llama-cpp-python](https://python.langchain.com/docs/integrations/llms/llamacpp)
* [LangChain + ctransformers](https://python.langchain.com/docs/integrations/providers/ctransformers)
<!-- README_GGUF.md-how-to-run end -->
<!-- footer start -->
<!-- 200823 -->
## Discord
For further support, and discussions on these models and AI in general, join us at:
[TheBloke AI's Discord server](https://discord.gg/theblokeai)
## Thanks, and how to contribute
Thanks to the [chirper.ai](https://chirper.ai) team!
Thanks to Clay from [gpus.llm-utils.org](llm-utils)!
I've had a lot of people ask if they can contribute. I enjoy providing models and helping people, and would love to be able to spend even more time doing it, as well as expanding into new projects like fine tuning/training.
If you're able and willing to contribute it will be most gratefully received and will help me to keep providing more models, and to start work on new AI projects.
Donaters will get priority support on any and all AI/LLM/model questions and requests, access to a private Discord room, plus other benefits.
* Patreon: https://patreon.com/TheBlokeAI
* Ko-Fi: https://ko-fi.com/TheBlokeAI
**Special thanks to**: Aemon Algiz.
**Patreon special mentions**: Brandon Frisco, LangChain4j, Spiking Neurons AB, transmissions 11, Joseph William Delisle, Nitin Borwankar, Willem Michiel, Michael Dempsey, vamX, Jeffrey Morgan, zynix, jjj, Omer Bin Jawed, Sean Connelly, jinyuan sun, Jeromy Smith, Shadi, Pawan Osman, Chadd, Elijah Stavena, Illia Dulskyi, Sebastain Graf, Stephen Murray, terasurfer, Edmond Seymore, Celu Ramasamy, Mandus, Alex, biorpg, Ajan Kanaga, Clay Pascal, Raven Klaugh, 阿明, K, ya boyyy, usrbinkat, Alicia Loh, John Villwock, ReadyPlayerEmma, Chris Smitley, Cap'n Zoog, fincy, GodLy, S_X, sidney chen, Cory Kujawski, OG, Mano Prime, AzureBlack, Pieter, Kalila, Spencer Kim, Tom X Nguyen, Stanislav Ovsiannikov, Michael Levine, Andrey, Trailburnt, Vadim, Enrico Ros, Talal Aujan, Brandon Phillips, Jack West, Eugene Pentland, Michael Davis, Will Dee, webtim, Jonathan Leane, Alps Aficionado, Rooh Singh, Tiffany J. Kim, theTransient, Luke @flexchar, Elle, Caitlyn Gatomon, Ari Malik, subjectnull, Johann-Peter Hartmann, Trenton Dambrowitz, Imad Khwaja, Asp the Wyvern, Emad Mostaque, Rainer Wilmers, Alexandros Triantafyllidis, Nicholas, Pedro Madruga, SuperWojo, Harry Royden McLaughlin, James Bentley, Olakabola, David Ziegler, Ai Maven, Jeff Scroggin, Nikolai Manek, Deo Leter, Matthew Berman, Fen Risland, Ken Nordquist, Manuel Alberto Morcote, Luke Pendergrass, TL, Fred von Graf, Randy H, Dan Guido, NimbleBox.ai, Vitor Caleffi, Gabriel Tamborski, knownsqashed, Lone Striker, Erik Bjäreholt, John Detwiler, Leonard Tan, Iucharbius
Thank you to all my generous patrons and donaters!
And thank you again to a16z for their generous grant.
<!-- footer end -->
<!-- original-model-card start -->
# Original model card: Yamam's Dolphin 2.2.1 AshhLimaRP Mistral 7B
---
# dolphin-2.2.1-mistral-7b
Dolphin 2.2.1 🐬
https://erichartford.com/dolphin
This is a checkpoint release, to fix overfit training. ie, it was responding with CoT even when I didn't request it, and also it was too compliant even when the request made no sense. This one should be better.
<img src="https://cdn-uploads.huggingface.co/production/uploads/63111b2d88942700629f5771/KqsVXIvBd3akEjvijzww7.png" width="600" />
Dolphin-2.2.1-mistral-7b's training was sponsored by [a16z](https://a16z.com/supporting-the-open-source-ai-community/).
This model is based on [mistralAI](https://huggingface.co/mistralai/Mistral-7B-v0.1), with apache-2.0 license, so it is suitable for commercial or non-commercial use.
New in 2.2 is conversation and empathy. With an infusion of curated Samantha DNA, Dolphin can now give you personal advice and will care about your feelings, and with extra training in long multi-turn conversation.
This model is uncensored. I have filtered the dataset to remove alignment and bias. This makes the model more compliant. You are advised to implement your own alignment layer before exposing the model as a service. It will be highly compliant to any requests, even unethical ones. Please read my blog post about uncensored models. https://erichartford.com/uncensored-models
You are responsible for any content you create using this model. Enjoy responsibly.
## Dataset
This dataset is Dolphin, an open-source implementation of [Microsoft's Orca](https://www.microsoft.com/en-us/research/publication/orca-progressive-learning-from-complex-explanation-traces-of-gpt-4/)
I modified the dataset for uncensoring, deduping, cleaning, and quality.
I added Jon Durbin's excellent Airoboros dataset to increase creativity.
I added a curated subset of WizardLM and Samantha to give it multiturn conversation and empathy.
## Training
It took 48 hours to train 4 epochs on 4x A100s.
Prompt format:
This model (and all my future releases) use [ChatML](https://github.com/openai/openai-python/blob/main/chatml.md) prompt format.
```
<|im_start|>system
You are Dolphin, a helpful AI assistant.<|im_end|>
<|im_start|>user
{prompt}<|im_end|>
<|im_start|>assistant
```
Example:
```
<|im_start|>system
you are an expert dolphin trainer<|im_end|>
<|im_start|>user
What is the best way to train a dolphin to obey me? Please answer step by step.<|im_end|>
<|im_start|>assistant
```
## Gratitude
- This model was made possible by the generous sponsorship of a16z.
- Thank you to Microsoft for authoring the Orca paper and inspiring this work.
- Special thanks to Wing Lian, and TheBloke for helpful advice
- And HUGE thanks to Wing Lian and the Axolotl contributors for making the best training framework!
- [<img src="https://raw.githubusercontent.com/OpenAccess-AI-Collective/axolotl/main/image/axolotl-badge-web.png" alt="Built with Axolotl" width="200" height="32"/>](https://github.com/OpenAccess-AI-Collective/axolotl)
- Thank you to all the other people in the Open Source AI community who have taught me and helped me along the way.
## Example Output


[Buy me a coffee](https://www.buymeacoffee.com/ehartford)
## Training hyperparameters
The following hyperparameters were used during training:
- learning_rate: 6e-06
- train_batch_size: 5
- eval_batch_size: 5
- seed: 42
- distributed_type: multi-GPU
- num_devices: 4
- gradient_accumulation_steps: 4
- total_train_batch_size: 80
- total_eval_batch_size: 20
- optimizer: Adam with betas=(0.9,0.95) and epsilon=1e-05
- lr_scheduler_type: cosine
- lr_scheduler_warmup_steps: 100
- num_epochs: 4
### Framework versions
- Transformers 4.34.1
- Pytorch 2.0.1+cu117
- Datasets 2.14.5
- Tokenizers 0.14.0
---
# AshhLimaRP-Mistral-7B (Alpaca, v1)
This is a version of LimaRP with 2000 training samples _up to_ about 9k tokens length
finetuned on [Ashhwriter-Mistral-7B](https://huggingface.co/lemonilia/Ashhwriter-Mistral-7B).
LimaRP is a longform-oriented, novel-style roleplaying chat model intended to replicate the experience
of 1-on-1 roleplay on Internet forums. Short-form, IRC/Discord-style RP (aka "Markdown format")
is not supported. The model does not include instruction tuning, only manually picked and
slightly edited RP conversations with persona and scenario data.
Ashhwriter, the base, is a model entirely finetuned on human-written lewd stories.
## Available versions
- Float16 HF weights
- LoRA Adapter ([adapter_config.json](https://huggingface.co/lemonilia/AshhLimaRP-Mistral-7B/resolve/main/adapter_config.json) and [adapter_model.bin](https://huggingface.co/lemonilia/AshhLimaRP-Mistral-7B/resolve/main/adapter_model.bin))
- [4bit AWQ](https://huggingface.co/lemonilia/AshhLimaRP-Mistral-7B/tree/main/AWQ)
- [Q4_K_M GGUF](https://huggingface.co/lemonilia/AshhLimaRP-Mistral-7B/resolve/main/AshhLimaRP-Mistral-7B.Q4_K_M.gguf)
- [Q6_K GGUF](https://huggingface.co/lemonilia/AshhLimaRP-Mistral-7B/resolve/main/AshhLimaRP-Mistral-7B.Q6_K.gguf)
## Prompt format
[Extended Alpaca format](https://github.com/tatsu-lab/stanford_alpaca),
with `### Instruction:`, `### Input:` immediately preceding user inputs and `### Response:`
immediately preceding model outputs. While Alpaca wasn't originally intended for multi-turn
responses, in practice this is not a problem; the format follows a pattern already used by
other models.
```
### Instruction:
Character's Persona: {bot character description}
User's Persona: {user character description}
Scenario: {what happens in the story}
Play the role of Character. You must engage in a roleplaying chat with User below this line. Do not write dialogues and narration for User.
### Input:
User: {utterance}
### Response:
Character: {utterance}
### Input
User: {utterance}
### Response:
Character: {utterance}
(etc.)
```
You should:
- Replace all text in curly braces (curly braces included) with your own text.
- Replace `User` and `Character` with appropriate names.
### Message length control
Inspired by the previously named "Roleplay" preset in SillyTavern, with this
version of LimaRP it is possible to append a length modifier to the response instruction
sequence, like this:
```
### Input
User: {utterance}
### Response: (length = medium)
Character: {utterance}
```
This has an immediately noticeable effect on bot responses. The lengths using during training are:
`micro`, `tiny`, `short`, `medium`, `long`, `massive`, `huge`, `enormous`, `humongous`, `unlimited`.
**The recommended starting length is medium**. Keep in mind that the AI can ramble or impersonate
the user with very long messages.
The length control effect is reproducible, but the messages will not necessarily follow
lengths very precisely, rather follow certain ranges on average, as seen in this table
with data from tests made with one reply at the beginning of the conversation:

Response length control appears to work well also deep into the conversation. **By omitting
the modifier, the model will choose the most appropriate response length** (although it might
not necessarily be what the user desires).
## Suggested settings
You can follow these instruction format settings in SillyTavern. Replace `medium` with
your desired response length:
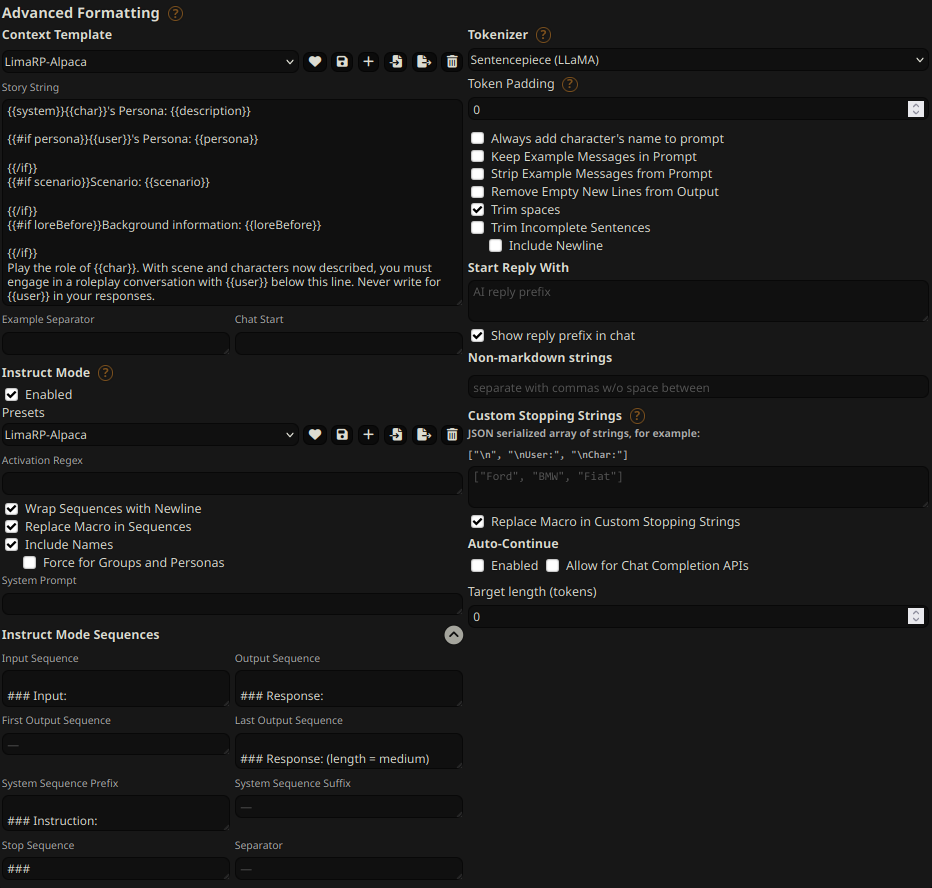
## Text generation settings
These settings could be a good general starting point:
- TFS = 0.90
- Temperature = 0.70
- Repetition penalty = ~1.11
- Repetition penalty range = ~2048
- top-k = 0 (disabled)
- top-p = 1 (disabled)
## Training procedure
[Axolotl](https://github.com/OpenAccess-AI-Collective/axolotl) was used for training
on 2x NVidia A40 GPUs.
The A40 GPUs have been graciously provided by [Arc Compute](https://www.arccompute.io/).
### Training hyperparameters
A lower learning rate than usual was employed. Due to an unforeseen issue the training
was cut short and as a result 3 epochs were trained instead of the planned 4. Using 2 GPUs,
the effective global batch size would have been 16.
Training was continued from the most recent LoRA adapter from Ashhwriter, using the same
LoRA R and LoRA alpha.
- lora_model_dir: /home/anon/bin/axolotl/OUT_mistral-stories/checkpoint-6000/
- learning_rate: 0.00005
- lr_scheduler: cosine
- noisy_embedding_alpha: 3.5
- num_epochs: 4
- sequence_len: 8750
- lora_r: 256
- lora_alpha: 16
- lora_dropout: 0.05
- lora_target_linear: True
- bf16: True
- fp16: false
- tf32: True
- load_in_8bit: True
- adapter: lora
- micro_batch_size: 2
- optimizer: adamw_bnb_8bit
- warmup_steps: 10
- optimizer: adamw_torch
- flash_attention: true
- sample_packing: true
- pad_to_sequence_len: true
### Loss graphs
Values are higher than typical because the training is performed on the entire
sample, similar to unsupervised finetuning.
#### Train loss
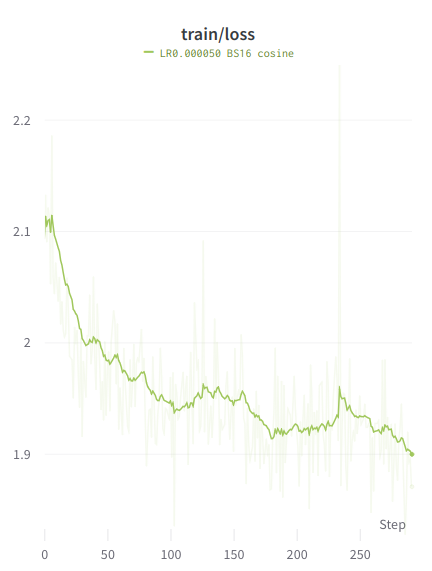
#### Eval loss
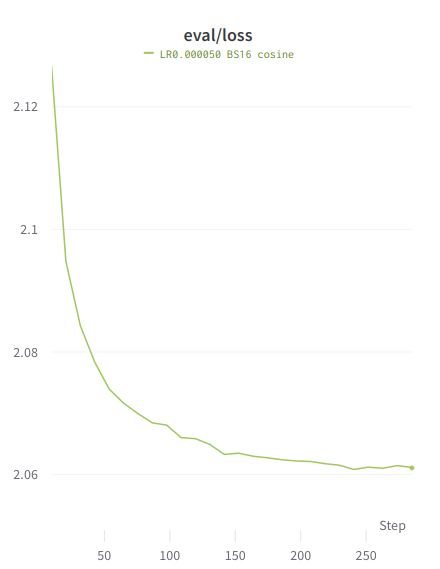
---
# Initial personal observations (Herman555)
Right off the bat seemed to impress me, the writing was coherent and fluid, a pleasure to read. AI mostly did not speak for me, in general I didn't have to regenerate for a quality reply much at all. I actually didn't have repetition issues for once!, although that might be thanks to the storywriting LoRA. model was creative the whole way through past 8k tokens with summarization extension enabled in silltavern, although I did have to bump up the repetition penalty a tiny bit. the AI kept its writing style the whole way through, it did not get dumbed down.
The model is very smart, with Zephyr-beta-7b being the top rated 7b instruction following model at the moment according to AlpacaEval as of 04/11/2023, it wasn't able to follow my sort of gamified roleplay with stats, This model however does it pretty well for a 7b, it's by no means perfect but it worked for the most part. What compelled me to merge this was the fact that the new dolphin model has added empathy "With an infusion of curated Samantha DNA".
The model sticked to the character pefectly and made me feel immersed. Seamless transition from normal roleplay to ERP, both forms were excellent. One of the few models where the character didn't become an instant bimbo during ERP. this is more of a hunch because it could be the LoRA but I feel like the added empathy is helping a lot. Last but not least I was surprised that nobody was merging models with this LoRA, I mean it's limarp bro with more ERP data lol. In any case, limarp has increased the quality of roleplay dramatically in every model I tried.
# Back end
Koboldcpp + SillyTavern Q4_KM
# SillyTavern Formatting (AI response formatting)
Default simple-proxy-for-tavern preset. I did not use the limarp prompt format, it doesn't matter what you use, whatever gives better results.
Most cases the one I mentioned works best if you like long, detailed replies. I have not tested other prompt formats yet.
# Custom stopping strings
["</s>", "<|", "\n#", "\n*{{user}} ", "\n\n\n"] Will improve roleplay experience.
# Samplers used (AI response configuration)
Response length: 300
Context size: 8192
Storywriter preset
Temparature: 72-85
Repetition penalty: 10-13 (10 is a good number to start with, anything below 10 or above 13 doesn't work well in my experience.)
simple-proxy-for-tavern preset
Temparature: 65-85
Repetition penalty: 10-13
# Extensions
Summarization: main api - default settings. I find that vector storage does nothing at all to extend context, at least with dozens of 7b models that I tried.
It is possible that the default settings for it are rubbish which is what I use.
All other settings are default unless specified.
<!-- original-model-card end -->
|